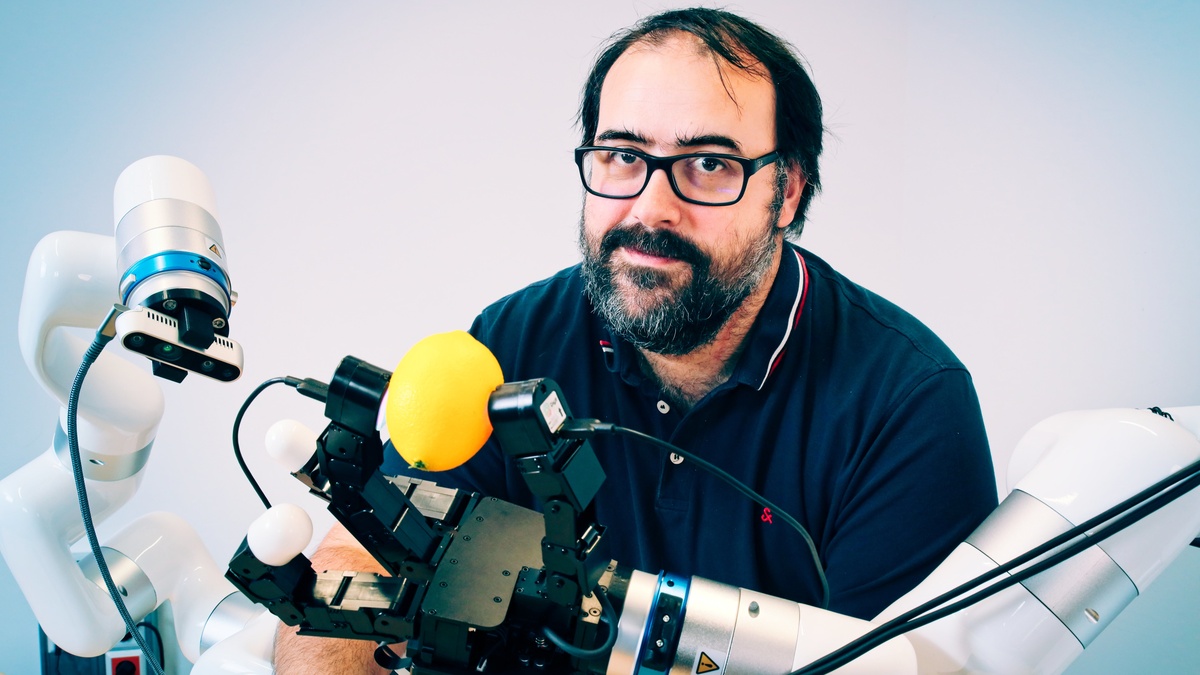
June 7, 2024
Prof Roberto Calandra is Honoured With the Early Academic Career Award in Robotics and Automation
The Early Academic Career Award recognises outstanding achievements in the area of the robotics and automation. This year, the award goes to Fellow Roberto Calandra for his significant contributions to touch sensing and processing and its application in manipulation. This recognition is given to academics who have made a noticeable and significant impact on the fields of robotics and automation through their work. Roberto Calandra, Professor of Explainable Artificial Intelligence at the Faculty of Computer Science, is honoured with the 2024 Early Academic Career Award in Robotics and Automation by the IEEE Robotics and Automation Society (RAS).
The award recognises the work he and his team have done in the field of touch sensor technology: from hardware design and software development to applications in the field of gripping and manipulation. One of their research focuses, in collaboration with Meta-Research Innovation Center at Stanford (METRICS) and the National Center for Tumour Diseases (NCT/UCC) Dresden, aims to find out how the use of touch sensors can help in the early detection of cancer. The scientists are developing robotic hands that can use vision-based tactile sensors to feel properties such as material type, shape, density and surface texture and achieve the strength and spatial resolution of human fingertips.
“I am very honoured to receive this award from the robotics community and to stand on the shoulders of the industry giants. As the sense of touch becomes increasingly important as a perceptual modality, we will continue to work on the democratisation of touch and its many applications,” emphasises Calandra.
About Roberto Calandra
Roberto Calandra holds the Chair of „Explainable Artificial Intelligence“ at TU Dresden and is the newest member of SECAI. His research aims to improve collaboration between humans and machines in real, virtual and remote environments. The professorship's research will contribute in particular to analysing learned models using machine learning methods such as deep learning, making statements and decisions made on the basis of these models explainable and developing new methods of explainability in machine learning.